
Generative AI has already begun to revolutionize the field of art and design by providing artists and designers with new tools and techniques for creating unique and personalized content. With the ability to generate new images, designs, and even entire environments, generative AI is opening up new possibilities for creative expression and innovation in the arts.
One area where generative AI is already having a significant impact is in the field of digital art. With the rise of generative art, artists are exploring new ways of creating interactive and immersive experiences that blur the lines between art and technology. Using techniques such as machine learning and computer vision, artists are able to create generative artworks that respond to the viewer and the environment in real-time, creating a dynamic and ever-changing experience.
Generative AI is also being used in the field of design to create new and innovative products and experiences. By leveraging generative design techniques, designers are able to create unique and customizable products that are tailored to the specific needs and preferences of individual users. For example, generative design can be used to create custom clothing, furniture, and even entire buildings that are optimized for specific use cases and environments.
Another area where generative AI is having a significant impact is in the field of music. With the ability to generate new melodies and harmonies based on existing musical patterns, generative AI is allowing musicians to explore new creative possibilities and push the boundaries of traditional music composition. By using generative AI tools, musicians are able to create complex and layered musical arrangements that would be difficult or impossible to create by hand.
Introduction
Artificial intelligence (AI) has revolutionized the way we approach many tasks in the modern world. From autonomous vehicles to voice assistants, AI has become an integral part of our lives. Generative AI is one of the most exciting developments in the field of AI. It is a subfield of machine learning that allows computers to generate data, such as images, videos, and even music, without explicit instructions from a programmer.
In this guide, we will explore the world of generative AI, including its definition, types, and applications. We will also discuss the importance of generative AI and the benefits it can provide to businesses. Finally, we will examine the risks associated with not adopting generative AI and provide recommendations for businesses looking to prepare for the future.
A Brief History of Generative AI
The history of generative AI can be traced back to the early days of artificial intelligence research in the 1950s and 1960s, when researchers began developing computer programs that could generate simple pieces of text or music. However, it wasn't until the rise of deep learning in the 2010s that generative AI began to make significant strides in terms of accuracy and realism.
One early milestone in the history of generative AI was the introduction of Experiments in Musical Intelligence (EMI) by David Cope in 1997. EMI was capable of generating new pieces of music in the style of famous composers using a combination of rule-based and statistical techniques.
Another milestone came in 2010, when Google introduced its "autocomplete" feature, which uses machine learning algorithms to predict what a user is typing and offer suggestions for how to complete the sentence. This feature is powered by a language model that has been trained on large amounts of text data, allowing it to generate plausible suggestions based on the context of the user's input.
In the same year, Apple introduced Siri, a voice assistant that was capable of understanding natural language queries and responding with relevant information or actions. Siri was an important milestone in the development of natural language processing (NLP) technologies, which are a key component of generative AI.
In 2013, a team of researchers at the University of Toronto led by Ruslan Salakhutdinov introduced the Deep Boltzmann Machine (DBM), a generative neural network that was capable of learning to represent complex distributions of data. This breakthrough paved the way for the development of GANs and other types of generative models.
One of the most important milestones in the history of generative AI came in 2014, when Ian Goodfellow and his colleagues introduced Generative Adversarial Networks (GANs). GANs are a type of neural network that can generate new data by pitting two networks against each other in a game-like setting. This breakthrough enabled the creation of realistic images and videos that could fool human observers.
In 2015, Amazon introduced Alexa, a voice assistant that could be integrated into various devices and respond to natural language queries. Like Siri, Alexa was an important milestone in the development of NLP technologies, and helped to popularize the use of voice assistants in daily life.
More recently, in 2019, OpenAI released its GPT-2 language model, which is capable of producing human-like text in a variety of styles and genres. This model was notable for its ability to generate long and coherent pieces of text that could easily pass as being written by a human.
Another important recent development in generative AI is the release of DALL-E, a neural network developed by OpenAI that can generate images from textual descriptions. DALL-E's ability to generate highly detailed and imaginative images has captured the public's imagination and sparked interest in the potential of generative AI.
Overall, the history of generative AI is a story of continuous innovation and breakthroughs in machine learning research. These early milestones demonstrate the progression of machine learning research towards increasingly sophisticated and realistic generative models. As the field continues to develop and new technologies are invented, we can expect to see even more impressive and creative applications of generative AI in the years to come.
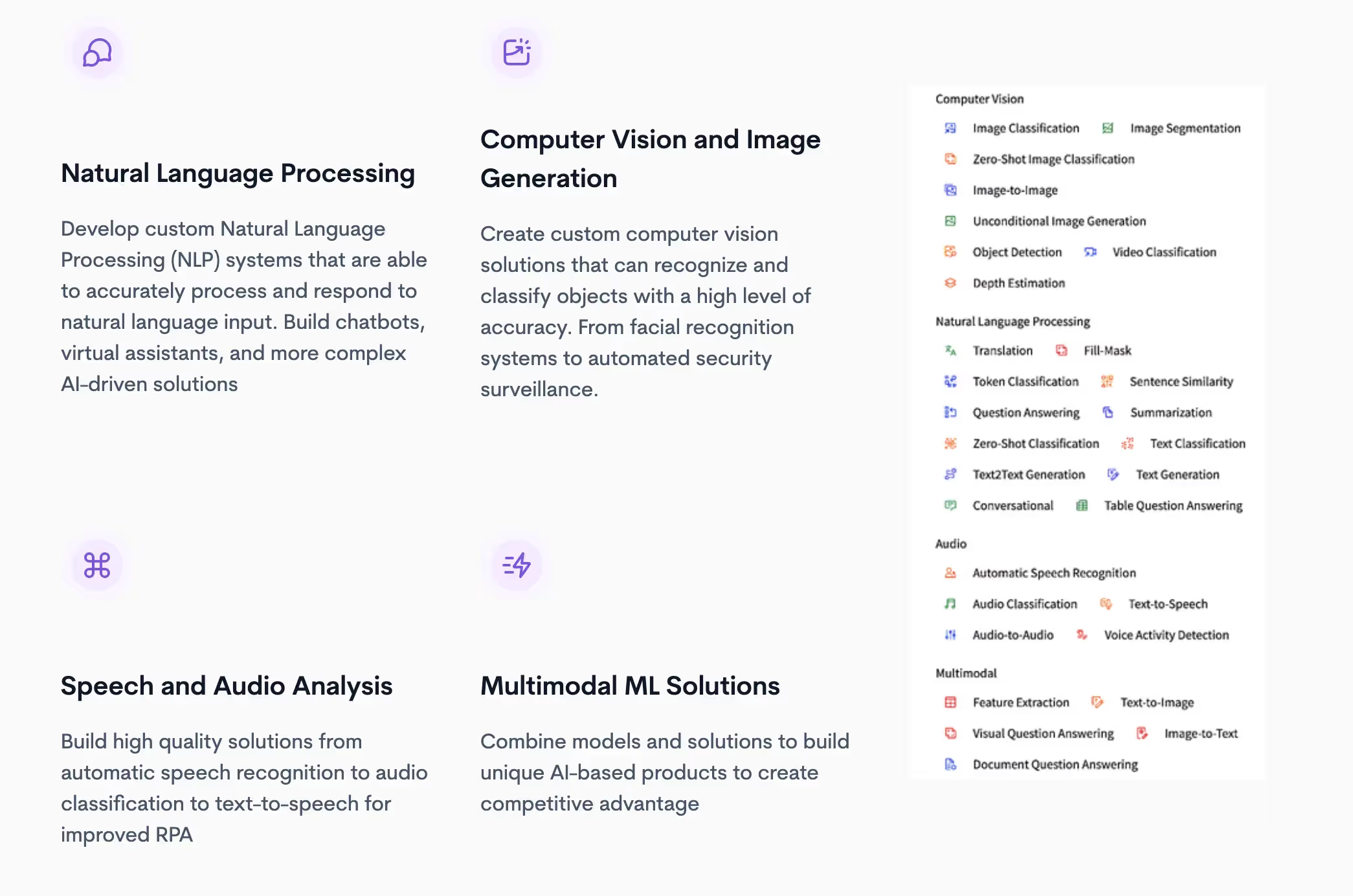
What is Generative AI
Generative AI is a subfield of machine learning that involves training computers to generate new data that is similar to data that it has been trained on. It is different from traditional machine learning, which involves teaching computers to recognize patterns in data and make predictions based on those patterns.
The main goal of generative AI is to enable computers to create new data that is realistic and similar to what a human might produce. This is done by training algorithms on large datasets to identify patterns and learn from them. Once the algorithm has learned the patterns, it can generate new data that fits the same patterns.
There are several types of generative AI, including generative adversarial networks (GANs), variational autoencoders (VAEs), and autoregressive models. Each type has its strengths and weaknesses and is suited for different applications.
Generative Adversarial Networks (GANs)
Generative adversarial networks (GANs) are a popular type of generative AI that involves two neural networks: a generator and a discriminator. The generator generates new data based on patterns it has learned from a training dataset, while the discriminator evaluates the generated data to determine if it is real or fake.
During training, the generator and discriminator play a game where the generator tries to generate data that is realistic enough to fool the discriminator, while the discriminator tries to distinguish between real and fake data. Through this game, the generator becomes better at creating data that is realistic and similar to the training dataset.
Variational Autoencoders (VAEs)
Variational autoencoders (VAEs) are another type of generative AI that involves encoding data into a lower-dimensional representation and then decoding it back into the original format. VAEs are similar to traditional autoencoders, but they introduce randomness into the encoding process, which allows them to generate new data that is similar to the training data.
During training, the VAE learns to encode the training data into a lower-dimensional representation, which can then be decoded back into the original format. The VAE can generate new data by randomly sampling from the lower-dimensional representation and decoding it back into the original format.
Autoregressive Models
Autoregressive models are a type of generative AI that involves predicting the probability of each element in a sequence based on the previous elements. Autoregressive models are commonly used in natural language processing and image generation.
During training, the autoregressive model learns to predict the probability of each element in a sequence based on the previous elements. The model can generate new data by predicting the next element in the sequence based on the previous elements and repeating this process until the desired length of the sequence is reached.
Potential of Generative AI
Generative AI has the potential to revolutionize the way businesses operate. It can be used to generate new designs, such as in the fashion industry, or to create new products, such as in the pharmaceutical industry. It can also be used to generate new content, such as in the gaming and entertainment industries.
By using generative AI, businesses can save time and money on product development and increase efficiency. Additionally, generative AI can help businesses stay ahead of the competition by allowing them to create unique products and content.
Overview of the Guide
In the following sections, we will delve deeper into the world of generative AI. We will explore the different types of generative AI and their applications, including deep learning, natural language processing, and computer vision. We will also examine real-world examples of generative AI and the risks associated with not adopting this technology.
It is important to note that while generative AI has many benefits, it also has its risks. In the final section, we will discuss these risks and provide recommendations for businesses looking to adopt generative AI.
By the end of this guide, you will have a better understanding of generative AI and its potential applications. You will also be equipped with the knowledge to make informed decisions about whether to adopt generative AI in your business.
As artificial intelligence (AI) continues to evolve and advance, one area of particular interest is generative AI. Generative AI is a type of AI that focuses on creating new data, such as images, text, and sound, rather than analyzing existing data. In this guide, we will explore the world of generative AI, including its definition, types, applications, and how it differs from traditional AI.
Section 1: Understanding Generative AI
Definition of Generative AI
Generative AI is a subfield of AI that focuses on creating new data that is similar to what a human might produce. Generative AI uses techniques such as deep learning, reinforcement learning, and evolutionary algorithms to create new data in a wide range of formats, such as images, text, and sound.
Types of Generative AI
There are several types of generative AI, including:
- Generative Adversarial Networks (GANs): GANs are a type of neural network that consists of two parts: a generator and a discriminator. The generator creates new data, while the discriminator evaluates the quality of the data. The two parts work together in a feedback loop to create high-quality data that is similar to what a human might produce.
- Variational Autoencoders (VAEs): VAEs are a type of neural network that are used for data compression and reconstruction. VAEs can also be used for generative tasks by sampling from the learned distribution to generate new data.
- Recurrent Neural Networks (RNNs): RNNs are a type of neural network that are designed to process sequential data, such as text or speech. RNNs can be used for generative tasks by training the network to predict the next character or word in a sequence.
Applications of Generative AI
Generative AI has many applications across a wide range of industries, such as:
- Art and Design: Generative AI can be used to create new art and designs, such as paintings, sculptures, and furniture.
- Entertainment: Generative AI can be used to create new music, movies, and video games.
- E-commerce: Generative AI can be used to create product descriptions and recommendations for online shopping.
- Medicine: Generative AI can be used to create new drugs and treatment plans based on patient data.
How it differs from Traditional AI
Generative AI differs from traditional AI in several ways. Traditional AI focuses on analyzing and processing existing data, while generative AI focuses on creating new data. Traditional AI is typically used for tasks such as classification, regression, and clustering, while generative AI is used for tasks such as image and text generation. Generative AI also requires significantly more computing power and data than traditional AI, due to the complex algorithms and models involved in creating new data.
In the following sections, we will explore each type of generative AI in more detail, including how they work and their specific applications. We will also discuss the potential benefits and risks of using generative AI, as well as the ethical and societal implications of this emerging technology.
Section 2: Deep Learning and Generative AI
Deep learning is a subset of machine learning that involves training neural networks with multiple layers to recognize patterns in data. Generative AI can also be achieved using deep learning techniques.
In particular, deep learning models such as convolutional neural networks (CNNs) and recurrent neural networks (RNNs) have been used in generative AI applications such as image and text generation.
Advantages and Disadvantages of Deep Learning
Deep learning has several advantages over traditional machine learning methods, including its ability to handle large amounts of data, its ability to learn hierarchical representations, and its ability to learn from unstructured data.
However, deep learning also has some disadvantages, including its high computational requirements, its reliance on large amounts of labeled data, and its tendency to overfit on training data.
Computational Requirements
The issue with high computational requirements is that it requires more computing power and data than traditional AI to process the complex algorithms and models needed for generative AI. This means that it is more expensive and time-consuming to build and run deep learning applications, which can be a barrier for some organizations. High computation requirements also limit the use of deep learning in environments with limited resources, such as mobile devices or edge computing systems. In these environments, streamlined algorithms may need to be used in order to reduce computational complexity. Ultimately, high computation requirements mean that not all organizations have access to these powerful tools due to hardware or software limitations.
Cloud to the rescue. Many cloud providers offer machine learning services that allow you to quickly and easily set up a deep learning model with minimal effort. For example, Amazon Web Services (AWS) offers Amazon Sagemaker, a fully managed service for training and deploying ML models which includes support for deep learning. Azure also offers support for deep learning with the Azure Machine Learning service. There are also a number of open source solutions, such as TensorFlow and PyTorch, that can be used to create and deploy deep learning models in the cloud.
Overfitting Risk
Overfitting occurs when a model has been trained too well on training data, resulting in poor performance on unseen test data. The result is a model that performs well on the specific dataset it was trained on, but does not generalize to other datasets or tasks. This can lead to inaccurate predictions and results. In order to avoid overfitting, it is important to use techniques such as regularization and cross-validation.
%20in%20Deep%20Learning.avif)
What are Generative Adversarial Networks (GANs) in Deep Learning
Generative adversarial networks (GANs) can be used in conjunction with deep learning techniques to create realistic images and other types of data. GANs can generate new data that is indistinguishable from real data, making them useful for applications such as image generation and video synthesis. Vendors and open source solutions such as StableDiffusion, Dall-e, or Generative Pre-trained Transformer (GPT) are available to enable GANs.
GANs can also be used to augment existing datasets, making them more diverse and reducing the need for additional labeled data. However, GANs can be difficult to train and may produce unrealistic data if not properly optimized.
How GANs work
Generative Adversarial Networks (GANs) are a type of neural network that consists of two parts: a generator and a discriminator. The generator creates new data, while the discriminator evaluates the quality of the data. The two parts work together in a feedback loop to create high-quality data that is similar to what a human might produce.
The generator starts by creating random noise, which is used as input. The generator then generates new data based on this noise. The discriminator evaluates the quality of the generated data and provides feedback to the generator. The generator then adjusts its parameters based on the feedback from the discriminator, and the process is repeated until the generated data is of high quality.
Applications of GANs
GANs have many applications across a wide range of industries, such as:
- Art and Design: GANs can be used to create new art and designs, such as paintings, sculptures, and furniture.
- Entertainment: GANs can be used to create new music, movies, and video games.
- Computer Vision: GANs can be used for tasks such as image and video generation, style transfer, and super-resolution.
- Medical Imaging: GANs can be used for tasks such as image segmentation and disease diagnosis.
Benefits and Risks of GANs
GANs have several benefits, including their ability to create high-quality data that is similar to what a human might produce. GANs can also be used for a wide range of applications, from art and design to medicine and science. Additionally, GANs have the potential to revolutionize industries such as entertainment and e-commerce by creating personalized content for users.
However, GANs also pose several risks, including their vulnerability to bias and errors. GANs are only as good as the data they are trained on, which means that if the data is biased or flawed, the generated output will be as well. Additionally, GANs can be used for malicious purposes, such as creating deepfakes or generating fake data to manipulate markets.
Ethical and Societal Implications of GANs
The use of GANs raises several ethical and societal concerns, such as the potential for misuse and the impact on employment. As GANs become more advanced and capable of generating high-quality data, there is a risk that they could be used for malicious purposes, such as creating deepfakes or manipulating markets.
Additionally, the use of GANs could have a significant impact on employment, particularly in industries such as art and design, where GANs could potentially replace human artists and designers. As with any new technology, it is important to consider the potential ethical and societal implications of GANs and to develop strategies to mitigate any negative effects.
How Recurrent Neural Networks (RNNs) Work
How RNNs work
Recurrent Neural Networks (RNNs) are a type of neural network that are designed to process sequential data, such as text or speech. RNNs can be used for generative tasks by training the network to predict the next character or word in a sequence.
RNNs work by maintaining an internal state, or memory, that is updated at each time step based on the input data and the previous state. The internal state allows the network to process sequential data and generate new data that is similar to the input data.
Applications of RNNs
RNNs have many applications across a wide range of industries, such as:
- Language Modeling: RNNs can be used for language modeling by predicting the next word in a sentence.
- Speech Recognition: RNNs can be used for speech recognition by predicting the next phoneme in a speech signal.
- Machine Translation: RNNs can be used for machine translation by predicting the next word in a translated sentence.
- Chatbots: RNNs can be used for chatbots by generating responses to user queries.
Benefits and Risks of RNNs
RNNs have several benefits, including their ability to process sequential data and generate new data that is similar to the input data. RNNs can also be used for a wide range of applications, from language modeling to speech recognition. Additionally, RNNs are more interpretable than other generative models, which can be beneficial in applications where understanding the underlying data is important.
However, RNNs also pose several risks, including their vulnerability to bias and errors. RNNs are only as good as the data they are trained on, which means that if the data is biased or flawed, the generated output will be as well. Additionally, RNNs can be used for malicious purposes, such as generating fake news or impersonating individuals.
Ethical and Societal Implications of RNNs
The use of RNNs raises several ethical and societal concerns, such as the potential for misuse and the impact on employment. As RNNs become more advanced and capable of generating high-quality data, there is a risk that they could be used for malicious purposes, such as generating fake news or impersonating individuals.
Additionally, the use of RNNs could have a significant impact on employment, particularly in industries such as customer service and journalism, where RNNs could potentially replace human workers. As with any new technology, it is important to consider the potential ethical and societal implications of RNNs and to develop strategies to mitigate any negative effects.
What is Reinforcement Learning
Reinforcement learning is a type of machine learning that involves training an algorithm to make decisions based on feedback from its environment. It is a key component of many generative AI applications, particularly those involving game playing and robotics. In these contexts, reinforcement learning can be used to teach an AI agent to generate actions that will maximize a reward signal, such as winning a game or completing a task.
One notable example of reinforcement learning in generative AI is the use of deep reinforcement learning to train game-playing AI agents, such as the AlphaGo system developed by Google DeepMind. AlphaGo used a combination of deep learning and reinforcement learning techniques to learn how to play the complex game of Go at a superhuman level.
Reinforcement learning also has potential applications in robotics, where it can be used to train robots to perform complex tasks such as object manipulation and locomotion. By learning from feedback signals such as visual feedback or haptic feedback, reinforcement learning algorithms can generate actions that allow a robot to perform a desired task. Read our Overview of Reinforcement Learning.
Overall, reinforcement learning is a powerful tool in the generative AI toolkit, with applications in a wide range of domains. As this field continues to evolve, we can expect to see further developments and applications of reinforcement learning in generative AI systems.
Section 3: Natural Language Processing (NLP) and Generative AI
Natural language processing (NLP) is a subfield of artificial intelligence that focuses on enabling computers to understand and interpret human language. NLP techniques can be used in generative AI to create new text and speech data, such as product descriptions, news articles, and synthetic voices for text-to-speech applications.
NLP Technologies in Generative AI
NLP technologies form the foundation of many generative AI applications. Some of the core NLP technologies used in generative AI include:
- Tokenization: The process of breaking down a text into individual units, or tokens, which can be words, punctuation, or other units depending on the application. Tokenization is the first step in many NLP tasks and is used to prepare text for further processing.
- Part-of-Speech (POS) Tagging: The process of labeling each word in a text with its corresponding part of speech, such as noun, verb, or adjective. POS tagging is used to analyze the grammatical structure of a sentence and is used in many NLP tasks, such as sentiment analysis and machine translation.
- Named Entity Recognition (NER): The process of identifying named entities in a text, such as people, places, and organizations. NER is used in many applications, such as search engines and chatbots, to extract relevant information from text.
- Sentiment Analysis: The process of determining the sentiment, or emotional tone, of a text. Sentiment analysis can be used to analyze customer feedback, social media posts, and other types of text data.
- Machine Translation: The process of automatically translating text from one language to another. Machine translation is a complex task that involves several NLP technologies, such as POS tagging and named entity recognition.
- Text Summarization: The process of generating a summary of a longer text. Text summarization can be done manually or automatically and is used in many applications, such as news and media.
Recent advances in deep learning have led to the development of neural NLP models, such as recurrent neural networks (RNNs) and transformer models like BERT and GPT-3, which have achieved state-of-the-art performance on many NLP tasks. These models have significantly improved the accuracy and efficiency of generative AI applications that rely on NLP technologies.
As we have discussed, RNNs are a type of neural network that are designed to process sequential data, such as text or speech. By maintaining an internal state, or memory, that is updated at each time step based on the input data and the previous state, RNNs are able to process sequential data and generate new data that is similar to the input data. RNNs have many applications across a wide range of industries, such as language modeling, speech recognition, machine translation, and chatbots.
Transformer models like BERT (Bidirectional Encoder Representations from Transformers) and GPT-3 (Generative Pre-trained Transformer 3) have taken NLP to the next level by leveraging the power of large-scale pre-training and fine-tuning. BERT, for example, is a transformer-based model that has achieved state-of-the-art performance on a wide range of NLP tasks, including sentiment analysis, named entity recognition, and question answering. GPT-3, on the other hand, is a massive transformer-based language model that has been pre-trained on a massive corpus of text and is capable of generating highly coherent and contextually relevant text.
- BERT (Bidirectional Encoder Representations from Transformers) was developed by researchers at Google AI Language in 2018. BERT is a transformer-based language model that is pre-trained on a large corpus of text data and can be fine-tuned for a wide range of NLP tasks, such as question answering, sentiment analysis, and named entity recognition. BERT has achieved state-of-the-art performance on many NLP tasks and has been widely adopted by researchers and industry practitioners alike.
- GPT (Generative Pre-trained Transformer) is a series of transformer-based language models developed by OpenAI. The first version of GPT, GPT-1, was released in 2018, and subsequent versions, GPT-2 and GPT-3, were released in 2019 and 2020, respectively. GPT-3, in particular, has generated significant interest and excitement in the AI community due to its massive size (175 billion parameters) and impressive performance on a wide range of NLP tasks.
- XLNet: A transformer-based language model that is pre-trained using a permutation language modeling objective and has achieved state-of-the-art performance on several NLP benchmarks.
- RoBERTa: A modified version of BERT that is pre-trained using a larger corpus of data and a more sophisticated pre-training process, resulting in improved performance on several NLP tasks.
- T5: A transformer-based model that is pre-trained on a wide range of NLP tasks and can be fine-tuned for various downstream tasks, including text classification and question answering.
- ELECTRA: A transformer-based model that is pre-trained using a novel pre-training objective called "discriminative masked language modeling" and has achieved state-of-the-art performance on several NLP tasks.
These neural NLP models have significantly improved the accuracy and efficiency of generative AI applications that rely on NLP technologies. For example, with the help of these models, generative AI can be used to generate new text data, such as product descriptions or news articles, with higher accuracy and relevance. Additionally, these models have enabled the development of more advanced chatbots and virtual assistants that can understand and respond to natural language queries with greater accuracy and efficiency
NLP Challenges in Generative AI
NLP poses several challenges in generative AI. One of the main challenges is the complexity of language. Language is highly nuanced and context-dependent, making it difficult for machines to fully understand and generate. In addition, language is constantly evolving, which means that NLP models need to be continually updated to reflect changes in language use.
Another challenge is the need for large amounts of labeled data. NLP models require labeled data to learn from, which means that generating high-quality data is crucial for the success of the model. However, generating labeled data can be time-consuming and expensive, which can limit the scalability of NLP models.
Use Cases of NLP in Generative AI
NLP has several use cases in generative AI. One of the most popular applications is chatbots, which use NLP to generate responses to user queries. Chatbots can be used in customer service, e-commerce, and other industries to provide 24/7 support to customers.
Another use case is text summarization, which involves generating a summary of a longer text. Text summarization can be used in news and media to provide quick summaries of news articles.
Future of NLP in Generative AI
NLP is a rapidly evolving field, and there are many exciting developments on the horizon. One area of research is neural machine translation, which involves using neural networks to translate text. Neural machine translation has shown promising results in recent years and could revolutionize the way we translate text.
Another area of research is natural language generation, which involves using NLP to generate new text that is coherent and meaningful. Natural language generation could be used in a wide range of applications, such as content creation, chatbots, and virtual assistants.
NLP is a powerful tool in generative AI that has many applications in text and speech generation. While NLP poses several challenges, including the need for large amounts of labeled data and the complexity of language, it has the potential to revolutionize the way we interact with machines and generate content. By leveraging NLP technologies and keeping up with the latest research developments, businesses can unlock new opportunities for innovation and growth.
Section 4: Computer Vision and Generative AI
Computer vision is a subfield of AI that focuses on enabling computers to interpret and understand visual data, such as images and videos. Computer vision can be used in generative AI to create new images and videos.
Applications of Computer Vision in Generative AI
Computer vision can be used to generate new images and videos, such as synthetic photographs or videos for special effects. Computer vision can also be used to augment existing images or videos, such as in the restoration of old photographs.
Synthetic Photographs and Generative AI
Generative AI has opened up a world of possibilities in creating highly realistic images and photographs that do not exist in the real world. By training deep neural networks on large datasets of images, and using those networks to generate new images that resemble those in the dataset, generative AI can create synthetic photographs that can be used in various industries.
One of the most promising applications of synthetic photographs generated by generative AI is in the e-commerce industry. They could be used to create highly realistic product images for online stores, reducing the need for expensive photo shoots. These synthetic photographs could be used to showcase a variety of products in different settings, allowing customers to get a better sense of the product before they buy it.
Another potential use case for synthetic photographs is in the entertainment industry. Generative AI can create highly realistic and detailed virtual environments for video games or for use in movies and TV shows. This can help to save time and money on creating physical sets or locations for filming.
The medical industry is another area where synthetic photographs generated by generative AI could have significant implications. Medical imaging is an area where there is a constant need for high-quality images, and generative AI has the potential to provide these images quickly and efficiently. Synthetic photographs generated by generative AI could be used to create highly detailed and realistic medical images that could aid in diagnosis and treatment planning.
Generative AI could also be used to create realistic images for use in architecture and interior design. Synthetic photographs could be used to create virtual tours of buildings or to showcase interior design ideas to clients.
Overall, the development of generative AI for the creation of synthetic photographs has significant potential for a wide range of industries. From e-commerce to entertainment, medicine to architecture, generative AI can create highly realistic images and photographs that can help businesses to save time and money while providing customers with better experiences.
From our perspective we believe the marketing agency market is ripe for disruption. Marketing agencies spend billions of dollars annually creating and recreating images to fit specific geographic markets. For instance the global coffee brand selling coffee across countries may want to target the audience in each region and present people enjoying their brand who look like their customers in that market. This small effort requires brands to recreate the same scenarios many times. With the advent of Generative AI, these brands may be able to shoot once and recreate the images to match their market. This saves on production, staff, models and more.
We believe Generative AI will have a huge impact on the marketing industry in the coming years. By utilizing generative AI and computer vision to create realistic synthetic photographs, marketers can reduce costs and increase efficiency. Furthermore, these synthetic images can be used across multiple markets, allowing for greater flexibility in targeting different audiences. We are excited to see how generative AI will continue to shape the marketing industry in the coming years.
Generative Models for Computer Vision
Generative models for computer vision include GANs and autoregressive models. GANs can be used to generate new images that are indistinguishable from real images, while autoregressive models can be used to generate new images by predicting the probability of each pixel based on the previous pixels.
Disadvantages of Computer Vision in Generative AI
While computer vision has many advantages in the realm of generative AI, there are also several notable disadvantages to consider. These include issues with unrealistic or distorted output, reliance on large amounts of labeled data, and potential for bias and discrimination in image recognition.
Unrealistic or Distorted Output
One of the main issues with computer vision is that it can produce unrealistic or distorted output. This can be due to a number of factors, including limitations in the algorithms used for image processing, or errors in the input data.
For example, some computer vision algorithms may have difficulty processing images with complex or highly detailed textures. This can result in distorted or unrealistic output, particularly when generating new images using generative AI techniques.
Another potential issue with computer vision is that it may produce output that is highly stylized or unrealistic. This can occur when the input data is biased or lacks diversity, leading to limited output options.
Reliance on Large Amounts of Labeled Data
Another major disadvantage of computer vision is its reliance on large amounts of labeled data. This can be a time-consuming and expensive process, particularly when working with complex image data.
To train computer vision models, data must be labeled with metadata such as object categories or image attributes. This requires a significant amount of human effort and expertise, making it difficult for small organizations or startups to develop computer vision applications.
Potential for Bias and Discrimination in Image Recognition
Perhaps the most concerning disadvantage of computer vision is its potential for bias and discrimination in image recognition. This occurs when computer vision algorithms are trained on biased or unrepresentative data, leading to inaccurate or discriminatory results.
For example, facial recognition algorithms have been shown to have lower accuracy rates for people with darker skin tones or for women. This is often due to biases in the data used to train the algorithm, which may be skewed towards certain groups of people.
Another example of bias in computer vision is in the realm of image labeling. If the data used to train an algorithm only includes images of men performing certain tasks, the algorithm may not recognize women performing the same tasks, leading to inaccurate results.
Technologies Contributing to Disadvantages in Computer Vision
There are several technologies that contribute to the disadvantages of computer vision. One example is transfer learning, which involves reusing pre-trained models for new tasks. While this can be an efficient way to develop computer vision applications, it can also result in biased or inaccurate results if the pre-trained models were trained on biased or unrepresentative data.
Another technology that can contribute to disadvantages in computer vision is deep learning, which involves training neural networks on large datasets of labeled data. While deep learning has revolutionized computer vision, it also requires significant amounts of labeled data and can be computationally expensive.
Mitigating the Disadvantages of Computer Vision
To mitigate the disadvantages of computer vision, several strategies can be employed. One strategy is to use diverse and representative datasets for training computer vision models, to reduce the potential for bias and discrimination.
Another strategy is to use algorithms and techniques that are robust to variations in input data, such as convolutional neural networks (CNNs). These algorithms can help to reduce the potential for unrealistic or distorted output in computer vision applications.
Overall, while computer vision has significant potential in the realm of generative AI, it is important to carefully consider its disadvantages and to develop strategies to mitigate these issues. By doing so, we can ensure that computer vision is used responsibly and ethically, and that it provides accurate and beneficial results for a wide range of applications.
Section 5: Variational Autoencoders (VAEs)
Variational Autoencoders (VAEs) are a type of generative AI model that can be used to generate new data points, such as images or music. VAEs are a type of neural network that can learn to represent complex data in a low-dimensional space, known as a latent space. This latent space can then be used to generate new data points that are similar to the original data.
In the context of generative AI, VAEs are used to generate new data points that are similar to the original data, but are not identical. For example, a VAE could be trained on a dataset of images of flowers, and then be used to generate new images of flowers that are similar to the original dataset, but are not exact copies.

VAEs work by encoding the input data into a low-dimensional latent space, and then decoding this latent representation back into the original data space. The encoder and decoder are trained together using a loss function that encourages the encoded representation to be meaningful and the decoded output to be similar to the original input.
One of the advantages of VAEs in generative AI is that they can generate a wide range of new data points, rather than simply copying or slightly modifying existing data points. This makes them useful for tasks such as image and music generation, where there is a high degree of variability in the data.
Another advantage of VAEs is that they can be used to generate data that is more diverse and novel than traditional generative models. By sampling from the latent space of a VAE, it's possible to generate new data points that are not present in the original dataset, but are still plausible based on the learned distribution of the data.
Applications of VAEs
VAEs have many applications across a wide range of industries, such as:
- Image Compression: VAEs can be used for image compression by encoding images into a low-dimensional representation and then decoding the representation back into the original image.
Speech Processing: VAEs can be used for tasks such as speech recognition, speech synthesis, and voice conversion.
- Recommendation Systems: VAEs can be used to generate personalized recommendations for users based on their past behavior and preferences.
- Drug Discovery: VAEs can be used to generate new drug candidates by encoding the chemical structure of existing drugs into a low-dimensional representation and then decoding the representation to create new drug candidates.
Benefits and Risks of VAEs
VAEs have several benefits, including their ability to compress and reconstruct data with high accuracy. VAEs can also be used for a wide range of applications, from image compression to drug discovery. Additionally, VAEs are more interpretable than other generative models, which can be beneficial in applications where understanding the underlying data is important.
However, VAEs also pose several risks, including the potential for overfitting and the difficulty of evaluating the quality of the generated data. VAEs can also be vulnerable to bias and errors if the training data is biased or flawed.
In short, VAEs are a powerful tool for generative AI, allowing for the creation of new data points that are similar to the original data, but not identical. By encoding data into a low-dimensional latent space, VAEs can generate a wide range of new data points that are diverse and novel.

Section 6: Real-World Examples of Generative AI
As we have covered in this guide, Generative AI has numerous applications in the real world, from creative industries to industrial and medical applications. In this section, we will explore some examples of how generative AI is being used today, as well as the advantages and disadvantages of these applications.
Creative Applications of Generative AI
Generative AI has significant potential for use in creative industries such as art, music, and fashion. By leveraging machine learning algorithms, artists and designers can create new and unique content that would be difficult or impossible to create manually.
For example, in the field of art, generative AI has been used to create new forms of digital art, such as deep dream images, which use convolutional neural networks to generate abstract and surreal images. In music, generative AI can be used to create new compositions or even generate entirely new musical styles.
In fashion, generative AI can be used to design new clothing styles or generate digital models that can be used in virtual try-on applications. By using generative AI to create new content, artists and designers can push the boundaries of creativity and create content that is truly unique.
Industrial Applications of Generative AI
Generative AI also has numerous applications in industrial settings, particularly in manufacturing and product design. By using generative AI to design products, engineers can create more efficient and cost-effective designs, while also reducing the time and resources required for product development.
For example, generative AI can be used to optimize the design of aircraft components, resulting in lighter and more fuel-efficient designs. In automotive manufacturing, generative AI can be used to design components such as engine parts or suspension systems, resulting in more efficient and reliable vehicles.
Medical Applications of Generative AI
Generative AI also has significant potential for use in the medical field, particularly in the areas of drug discovery and medical imaging. By using generative AI to analyze medical data, researchers and doctors can gain new insights into disease and develop more effective treatments.
For example, generative AI can be used to design new drugs by analyzing the chemical properties of existing drugs and generating new chemical structures that may be more effective. In medical imaging, generative AI can be used to analyze images such as X-rays or MRI scans, helping doctors to identify potential health issues more quickly and accurately.
Financial Services
Generative AI has numerous applications in the financial services industry, particularly in the areas of risk management and fraud detection. By using generative AI to analyze financial data, banks and other financial institutions can gain new insights into customer behavior and identify potential risks and fraudulent activities.
Hedge funds can leverage the processing power of generative AI to quickly analyze large financial data sets and extract key insights. This allows them to benchmark against comparables and make faster, data-driven decisions. With the help of intelligent applications, hedge funds can generate incisive reports in a matter of minutes, reducing the need for traditional financial analysts who rely on manual data processing. Ultimately, this could mark the end of the "models and bottles" financial analyst era, as generative AI solutions enable faster and more accurate decision-making.
Chatbots and Voicebots
Generative AI can also be used to develop chatbots and voicebots, which are computer programs designed to simulate conversation with human users. Chatbots and voicebots can be used in a wide range of applications, including customer service, marketing, and personal assistants.
Chatbots can be used in e-commerce to provide customers with information about products, help them make purchasing decisions, and provide support after a purchase has been made. Voicebots, on the other hand, can be used to provide hands-free assistance in environments such as cars or homes.
Section 7: Generative AI Disrupts the Status Quo
Generative AI has the potential to transform numerous industries by enabling faster, more accurate, and cost-effective decision-making. However, as with any technological disruption, it also has the potential to threaten traditional industries and job roles.
One area where generative AI could have a significant impact is in the field of SEO copywriting. By using natural language processing techniques, generative AI can be used to create unique, high-quality content at scale, reducing the need for human copywriters. This could have significant implications for the copywriting industry, which has traditionally relied on human writers to create content for websites, blogs, and other online platforms.
Another area where generative AI could have an impact is in back-office management. By automating tasks such as data entry and processing, generative AI can reduce the need for human workers in these roles. This could result in significant cost savings for businesses and organizations, but could also lead to job losses in the back-office management sector.
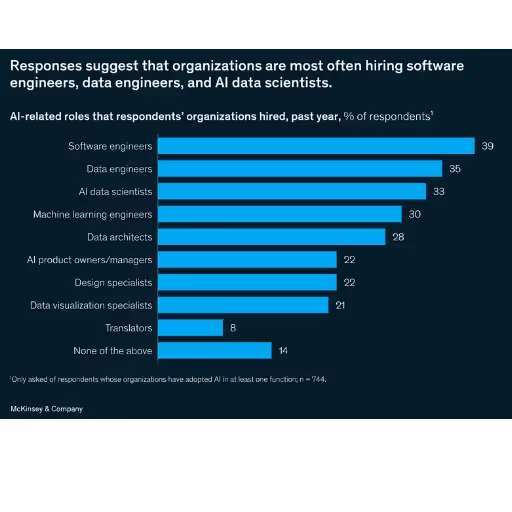
Generative AI could also have an impact on the legal industry, particularly in areas such as contract drafting and tax advice. By using machine learning algorithms to analyze legal documents and tax codes, generative AI could be used to create customized legal documents and tax advice at scale, reducing the need for human lawyers and accountants. This could have significant implications for the legal and accounting industries, which have traditionally relied on human experts to provide these services.
However, it's important to note that while generative AI has the potential to transform these industries, it's unlikely to completely replace human workers. Instead, generative AI is likely to augment human capabilities and allow workers to focus on higher-level tasks that require human judgment and creativity. Additionally, the accuracy and effectiveness of generative AI outputs will depend on the quality of the data used to train the models, as well as the domain-specific context provided.
Generative AI has significant potential to transform numerous industries, but also has the potential to threaten traditional job roles. As the technology continues to improve and becomes more context-specific, it will likely improve the accuracy and effectiveness of its outputs, leading to further disruption and transformation of industries. It's important for businesses and organizations to embrace generative AI as a tool for innovation and efficiency, while also considering the potential impact on workers and job roles.
Risks Associated with Not Adopting Generative AI
While generative AI has many benefits, there are also risks associated with not adopting this technology. Some of the risks include:
Falling Behind the Competition
Businesses that do not adopt generative AI risk falling behind their competitors, who may be using this technology to create new products and content more efficiently.
Inefficiency
Businesses that do not adopt generative AI risk being less efficient than their competitors, who may be able to generate new designs and products faster and with less cost.
Missed Opportunities
Businesses that do not adopt generative AI risk missing out on opportunities to create new products and content that could be highly profitable.
Conclusion
Generative AI is a powerful subfield of machine learning that has the potential to revolutionize the way businesses operate. By enabling computers to generate new data that is similar to what a human might produce, generative AI can be used to create new designs, products, and content. There are several types of generative AI, including GANs, VAEs, and autoregressive models, each suited for different applications. However, there are also risks associated with not adopting generative AI, including falling behind the competition, inefficiency, and missed opportunities. By considering these factors, businesses can make informed decisions about whether to adopt generative AI in their operations.
At Azumo, we have a long history building intelligent applications and solutions leveraging artificial intelligence and machine learning. If you are looking for developers who can support the build of your solution contact us today!
Try our AI-Powered Enterprise Search Solution.