.jpg)
Some people say agency is the defining attribute of video games as a language and as a medium. It’s what makes it stand out from other art and entertainment languages like film, TV, comics, and literature.
We can understand agency as the ability to act in a determinate environment and impact the surroundings.
So how do we use agency as a scope in game development? Ideally, by giving the player as many possibilities as we can, so they are able to interact with the environment in the most personalized and custom way possible. We can do that by giving the player a wide set of tools with very different uses, by creating complex NPC (enemy and non-enemy) AI, by using Nature Simulation Systems, among others.
Disclaimer: We understand this is not the only way to work with agency, (The Last of Us 2 does amazing things making you feel guilty for your actions while giving you very limited possibilities in terms of the outcome of those actions) but it’s an exceptionally useful tool for us to handle, both in the design and engineering sides of game development.
The idea of Nature Simulation Systems is not necessarily a new one, although there doesn’t seem to be a wide agreement of sorts to embrace these concepts under a single name. One popular source is The Nature of Code, the book written by processing YouTube star Daniel Shiffman. Most of what I’ll be talking about in this article can be accomplished following the book’s instructions.
Nature, as you might already know, works in infinitely unpredictable and emergent ways. So much that we might never “finish” studying it, even if we would be able to live forever. One of the aspects that interests us here is how a lot of times, simple behaviors can result in increasingly complex systems when interacting with other agents in a suitable environment. There might be thousands of different examples one can think of this principle, but there are some that have been researched and simulated in computers in several ways, for different purposes, and some of those are the ones we’ll tackle in this article.
Nature Simulation Systems
Cellular Automata
Cellular Automata (CA), at its core, might be the simplest complex system around. It is a system composed of individual elements that are called Cellular Automaton (or cell), which are as simple by themselves as a single bit.
A CA system consists of a grid where the cells “reside”. This grid can have any number of dimensions. We’ll think of a one-dimensional example right now to keep it simple.
Each cell has a state. The number of possible states can also vary in any number of possibilities we might think of. Again, we’ll keep it simple and imagine a cell with only two possible states (like a bit!).
Also, each cell has a neighborhood, which is basically a list of the cells near this one. Of course, this can also vary, for example setting the neighborhood to be the cells that fall inside a certain radio.
Finally, the cells in a CA we’ll have a ruleset that will dictate how their state will change over time according to their current state and their neighbors’ current state.
Now that we know the basic characteristics of how CAs work, we can look at how these rules can create complex behaviors. Wolfram Elementary CA is a pretty famous example that uses the simplest form of rules possible: a one-dimensional grid, two possible states, and only recognizes the immediately adjacent cells as neighbors. Using this starting point, we can now think of the possible rules we can give the cells. In this case there are exactly 256 possible rulesets.
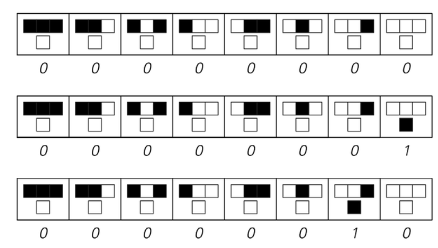
If each “generation” is visually shown below the previous one we can see how different patterns arrive.
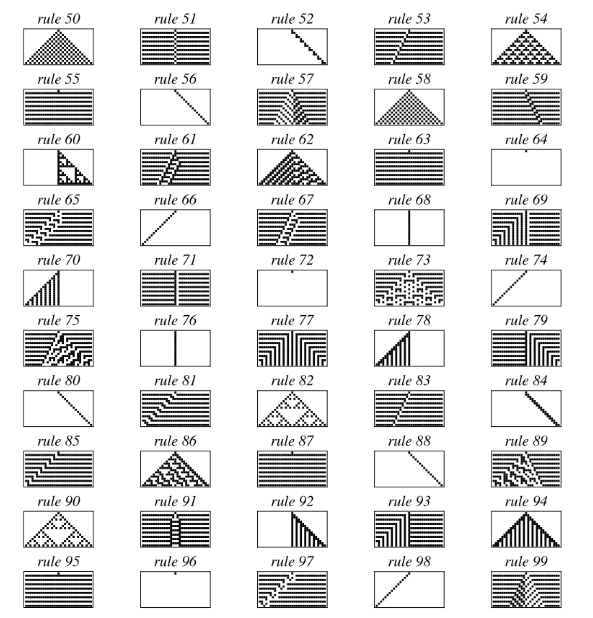
Now that we know how a CA works, we can imagine how much complexity can arise by simply changing the number of dimensions, states or neighbors.
The CA principle is often used in computer science for a lot of use cases, like bacteria behavior simulation and biology simulations in general, even anthropologic simulations. It’s even used outside of computer science for stuff like knitting!
Now, how does this apply to video games? There are a lot of ways CA can be used in gamedev, one of the most popular ones can be procedural generation (terrain, agents, treasure). There are even games that are almost completely a CA system (see Dwarf Fortress).
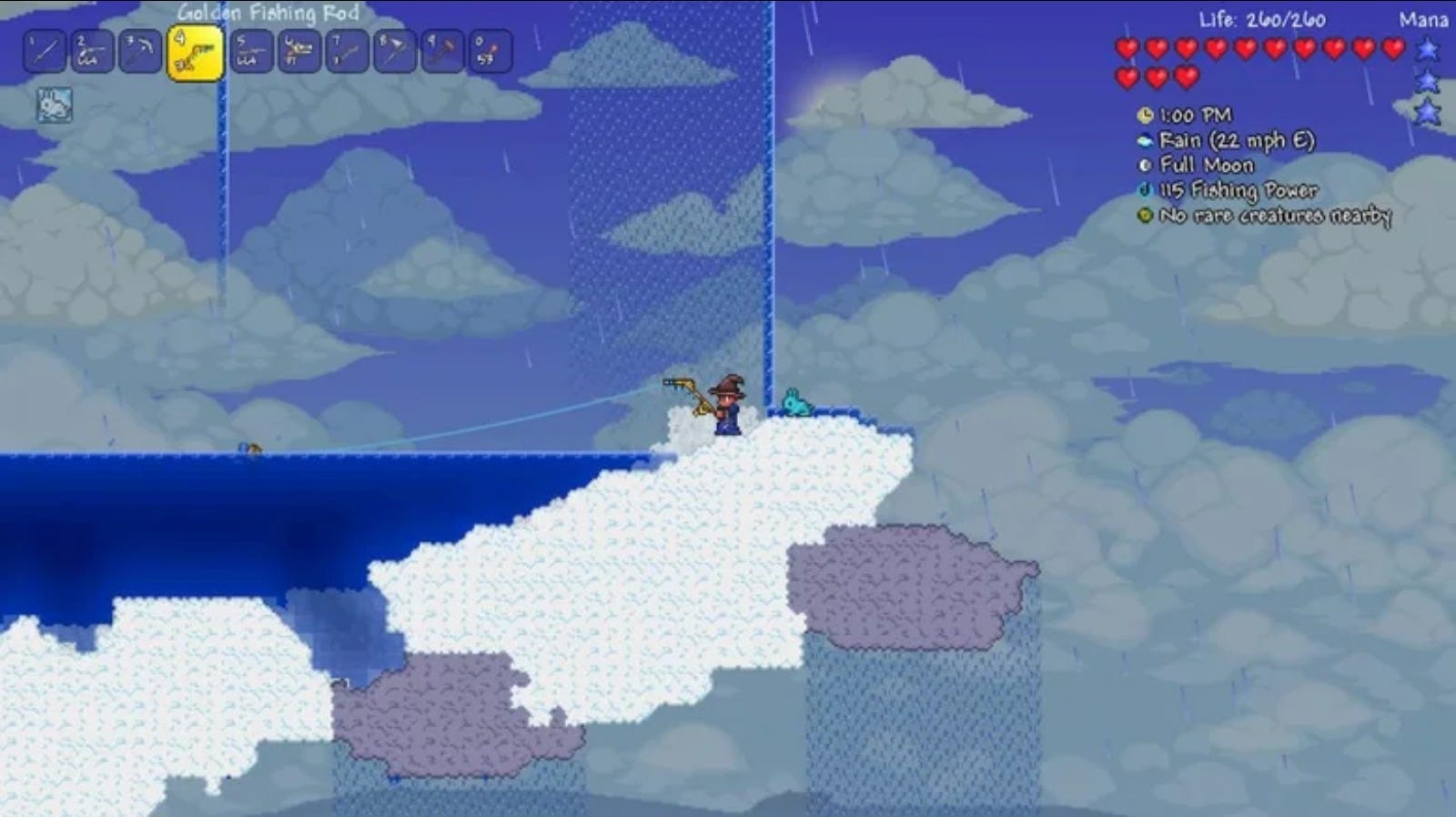
Fractals
Probably the most popular one, fractals are often used to create computer-generated images. We can see it in trippy electronic parties’ visuals, movies’ VFX, and digital art. Remember the visuals when playing music on WinAmp or the old Windows Video Player? Those where it seems we’re zooming in on abstract shapes endlessly but the shape always kind of looks the same from our point of view even though we zoomed in forever. That is a fractal.
So what does a shape need to be considered a fractal (and how can we create one)? First of all, fractals are -as mentioned in the example in the paragraph above- self similar. A smaller portion of the shape still looks like the bigger portion.
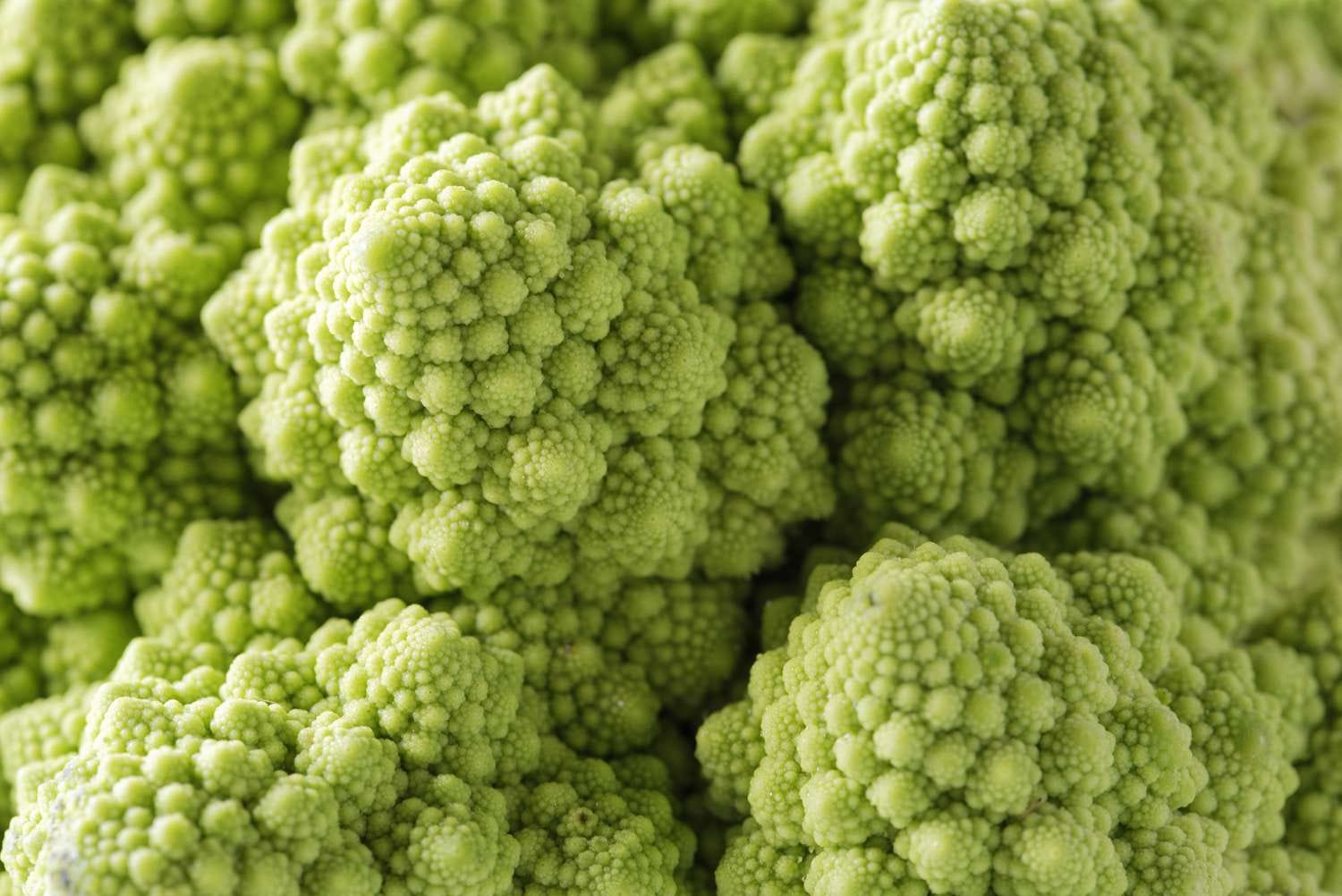
Another key aspect of a fractal is recursion, which means that the set of rules or algorithms we use to create a fractal at some point contains a reference to that same set of rules. One can imagine right away that with recursion we can create a function that does not explicitly show repetition but can create infinite outputs out of a finite statement. Of course, in programming, for that, we need an exit condition, a way to stop the recursion.
int fac1(int n) {if (n <= 0)return 1;elsereturn fac1(n-1)*n;}
A simple example of a recursive function.
Finally, one last aspect we need to take into consideration about fractals is that they don’t need to be “perfect”. Fractals come in two categories. A fractal whose smaller portion looks perfectly equal to its greater portion is known as deterministic; while a fractal where we don’t see exactly the same at different scales, but there’s a general quality of the shape’s structure and pattern is known as stochastic and it’s the one we’ll most likely see in nature.

Now we know what we need to create fractals, how do we use this in video games? Once again, one of our main use cases is procedural generation: probably every tree generator out there uses fractals to create the trees. They are also often used for VFX but usually in a non-interactive way (like a FMV scene of a character traveling to a different dimension and such). The truth is that, while fractals are a potentially huge tool, it can be very tricky to actually implement (both in the engineering and design sides): being able to create fractal interactive spaces or gadgets that don’t break or consume all our memory and, at the same time, are actually fun and rich to interact with can be an almost herculean task. Nonetheless, there are devs out there slaying the Nine-Headed Hydra: the trippy shooter Spaceflux and the Super Monkey Ball-esque racing game Marble Marcher are some pretty interesting examples to go check on.
Genetics
Genetics might be at the same time both the trickier system in this article (programming-wise) and the most used right now in computer science, with the rise of AI.
Genetic algorithms are models of simulations of evolution in software. Evolution in the terms we understand is based on the core principles described by Charles Darwin in The Origin of Species: over a number of generations, a population of entities/elements/objects evolve according to the principles of natural selection. Survival of the fittest and all that.
Let’s say we have a given problem, like “find the optimal route to go from point A to B”, and we have certain conditions to recognize a given route as optimal (short, safe, fun, etc.). Each of these entities can be seen as a possible solution to this problem.
We first create an initial generation of solutions, with a certain degree of variation on each of its parameters. Then there’s a stage of selection where we choose the best entities of the batch so they can “mate” to create a new generation. We do the selection by calculating the fitness of the entities, as to check who will be the fittest to survive. A disclaimer to be signed here is that we might instinctively think that we need two entities to create a new batch, like how it happens with animals, but here we can choose any number of survivors to mate (one, three, ten).
With the “parents” selected comes the reproduction stage, where we mix their genetic information to create the children. We do this by first making a crossover of the data, which can be performed in any way we might want (use the first half of one parent’s data and the second half of the other, mix it randomly, etc) and then adding a certain degree of artificial mutation to the mix. Why? Because there always might be a certain part of that “optimal” answer we are looking for that is not present in any of the selected entities, so randomizing a small fraction of that data can help us get that.
The exit state of our algorithm will then be to get an optimal solution, or in some cases the closest we can get to optimal by a given rate.
So how about video games? A quite popular (and literal) approximation to genetics in video games is the game Spore developed by Will Wright’s studio Maxis (probably the kings of emergent gameplay during the 90’s and 00’s). Besides a few examples like that, there aren’t a whole lot of games where we can see genetics in action; but it can be a useful tool during development nonetheless. AI training for enemies and NPCs in general can be done a lot faster and more elegantly with the use of genetic algorithms. We can even make the enemies learn throughout the game to exploit each individual player’s weaknesses in theory -EA allegedly did that with FIFA 22-.

Flocking Systems
The final system we’ll tackle in this article are Flocking Systems. Flocking systems are really useful for simulation large groups of simple agents that move through space. Flocks of birds, schools of fishes, swarms of bugs, herds in general. They are, at its core, complex systems: systems composed of many components that interact with each other. They are, as a whole, more than the sum of their parts. Usually, the individual components -the simple part of the complex whole- are agents with basic behaviors that, when interacting with a large number of pairs, can create complex and unpredictable results that blend between order and chaos.
The individual autonomous agent usually has three basic parameters (besides some basic stuff like move speed and rotation speed): separation tells the agent to avoid bumping into any of its pairs; alignment dictates the actual direction the agent wants to follow, which will be decided according to the general direction its neighboring pairs are taking; and cohesion which means that the agent will group with its neighbors. Each of these parameters also has a weight which basically gives us a priority order on how to mix them into one single desired speed and direction.
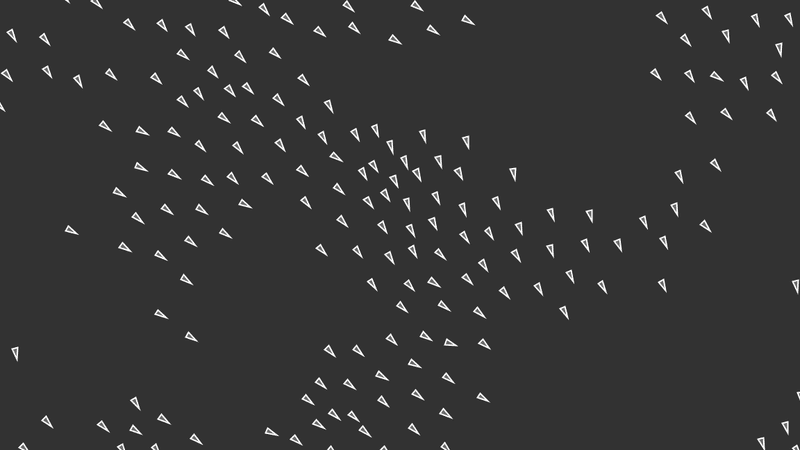
Simply messing around with these three basic parameters we can get lots of different behaviors for our system. Additionally, we can create stuff like a seek parameter and a fear parameter. The first one is to give the agents a final goal, besides their short-term goals of moving together. The second one can be used to make the agents avoid certain areas or objects in the environment. With these parameters, we can get a lot more control over our system when we don’t want the agents to wander aimlessly into the horizon.
So again, video games. Flocking systems usually create behaviors that are pretty to look at, although they are not usually super used in terms of gameplay. Probably every AAA out there uses flocking to simulate some group of animals or bugs that move in an ordered but emergent fashion. We at Azumo are currently working on a little video game project tentatively called Flock Hunt, where you get the role of an animal control employee in the town of Zumo, who has to capture a bunch of sick birds for your office to heal them. The birds as a group function as (you guessed it) a flocking system.
The birds use a fear parameter to avoid bumping into the limits the designer creates, so they won’t go too far away. Also, we have different species of birds, and each species has its own weight to their alignment, separation, and cohesion parameters (i.e. each species has a slightly different behavior). Mixing different species at the same level can create increasingly complex situations for the player to take into account. All of this by simply changing the value of some basic parameters.
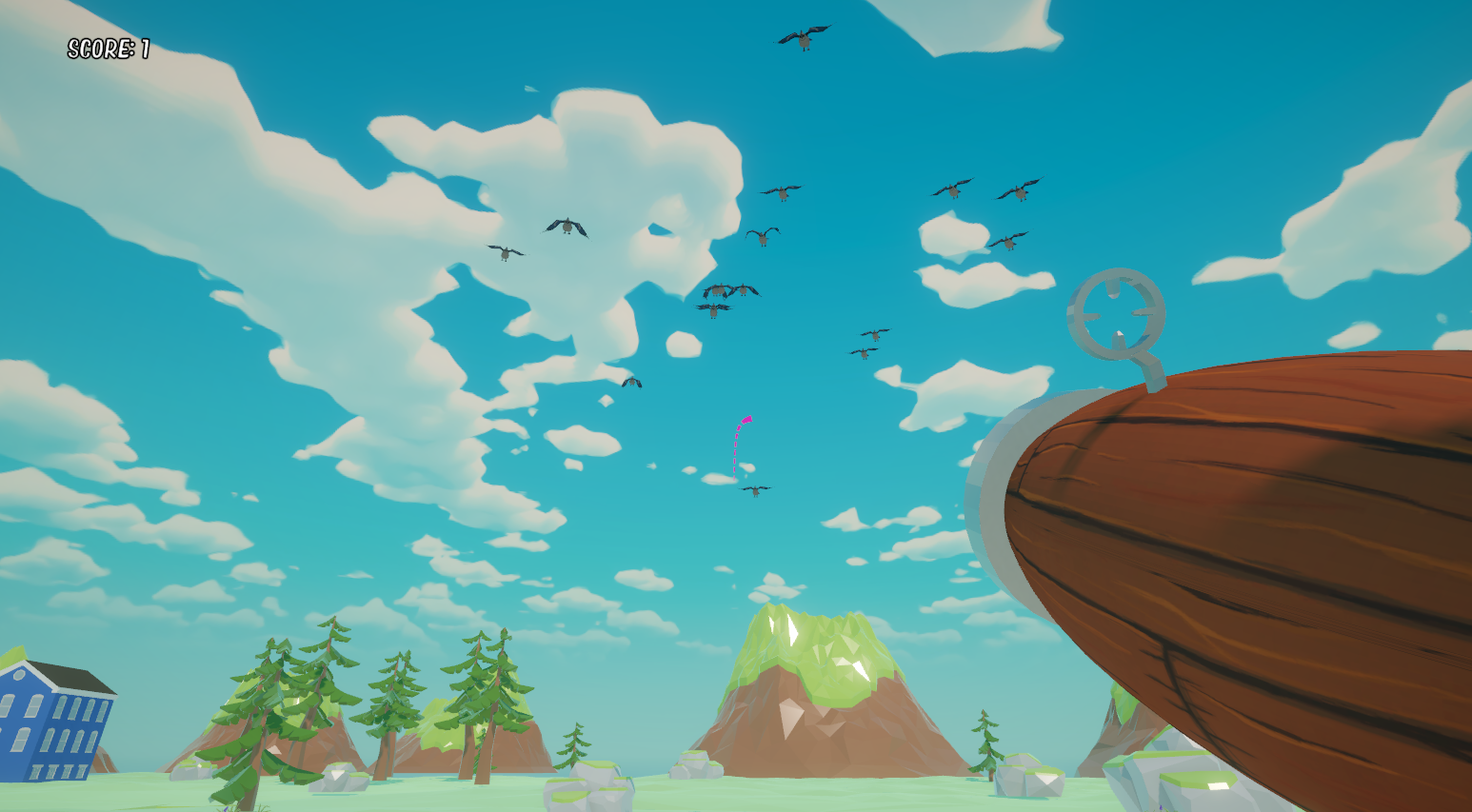
Closing words
Of course, in this article, we merely scratched the surface of some nature simulation systems just to get an overview of what is possible in video games with these tools, the possibility space will potentially increase as you dig deeper. Emergence is one of the things gamers cherish the most (even though they might not even know it) so I hope this approximation to nature simulation systems will be helpful to create richer gaming experiences.